Play by Play: Choosing a Product Adoption Metric
In today's business environment, companies must make swift and accurate decisions to stay competitive. At Convictional, we're dedicated to enhancing decision making by applying our software and AI tools. Historically, our decisions involved a mix of discussion and data analysis—a method common across the tech industry, regardless of company size. As we have developed our tool, we have been evolving the methods that we use to make decisions, to consider context more broadly and collaborate more intentionally.
As we have been working on decisions, we realized that there are no established norms for how to assess product adoption. We want customers to adopt our product so we can learn. We had to engage in a high stakes decision to choose a metric to use. If we chose wrong, we would emphasize the wrong product development bets for as long as that metric was in effect, potentially six months or longer.
As a founder, I often prefer intuitive, quick decisions—a concept psychologist Daniel Kahneman describes as 'System One' thinking. While this approach can be efficient, it sometimes lacks the thorough analysis provided by 'System Two' thinking, which is slower and more deliberate. Most decisions I made historically were driven by my initial hunch rather than a rigorous decision process.
My hunch in this case was to use the number of decisions customers completed per week in the app:
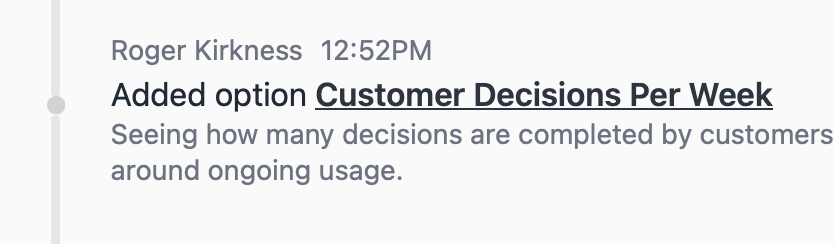
Despite my strong initial hunch about which metric to choose, I decided to 'dogfood'—use our product internally—to guide this critical decision. I realized that relying on my intuition might not be sufficient. By trusting the process and our tool's capabilities, I opened the door to potentially better outcomes. Interestingly, we later discovered that approximately 80% of the decision criteria generated in our software came from AI brainstorming rather than human input. This highlighted the tool's strength in formulating considerations.
I started by describing the nature of the decision I was trying to make:
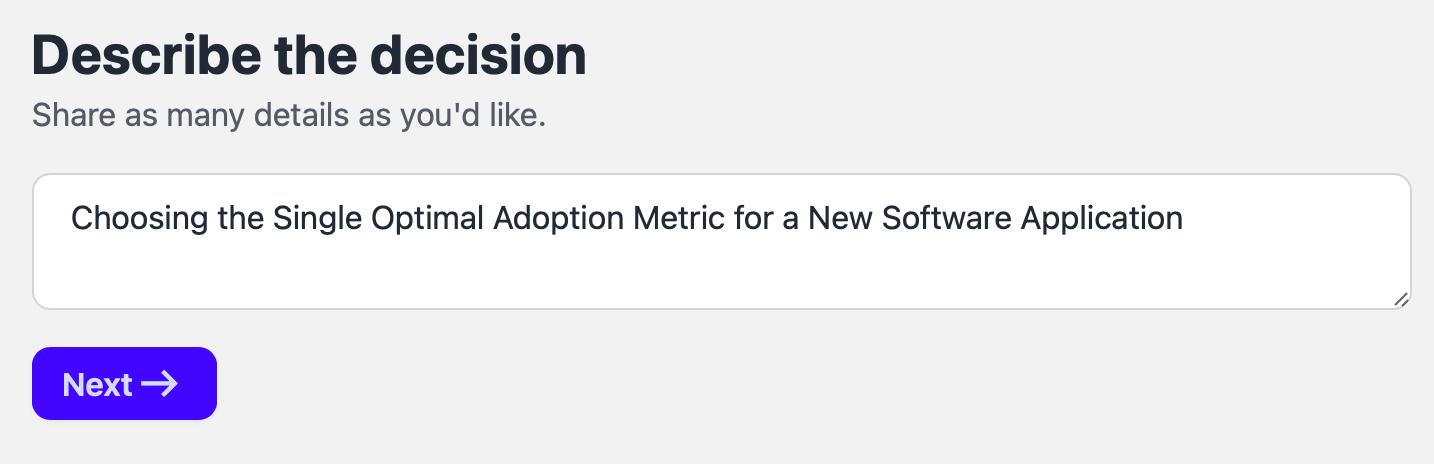
After that, I entered my goal. Overall my goal was to choose the right metric that would allow us to assess the progress we are making as we launch the research version of our product. Thinking about what your goal is when making a decision is a healthy decision hygiene step that I had made a habit of during prior iterations of our decision process, and confirmed by our academic advisors:

I also established a due date of two days from the creation date of the process, which was aggressive. This was partly because I was emotionally eager to finalize a metric so that I could communicate it to the team and filter and align our R&D efforts. The decision ended up taking almost a week to finalize, so my initial due date was blown because of how the process evolved each day.
Once I had created a decision in our tool with the right outline, I worked on adding people to the process. There are a few people on the team that I trust the most to weigh in on strategy decisions, and a few more I added for their analytical perspective after the process was in motion. The tool recommended the right people initially based on other decisions I’ve worked with them on in the past:
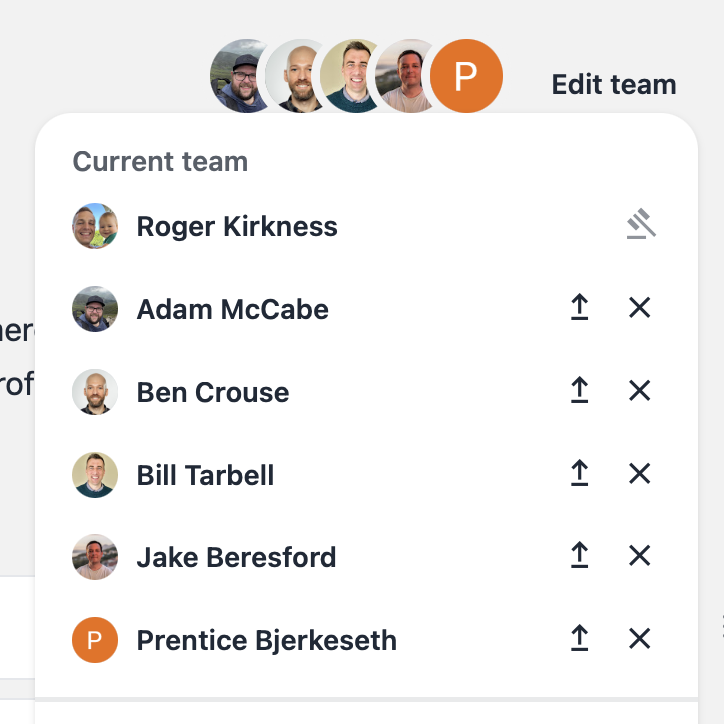
It can be quite challenging to know who to involve in a decision initially. One thing we’ve learned from our academic advisors is that collaborative decisions tend to have less extreme outcomes than decisions taken alone. Which means the more people you include, the more you’re going to be getting a considered but perhaps less exceptional outcome. For adoption metrics, I felt this was appropriate.
Bill on our team started to help shape the process the moment he joined. For example, he emphasized and deemphasized certain criteria and options that I had used our tool to brainstorm, as well as offering his input on the options I had initially proposed:

Without Bill’s input I wouldn’t have considered the importance of assessing not only how many decisions users are making, but also whether they are making them as a team or as an individual. For the reasons above, we ultimately want the latter. After sleeping on the team’s initial reactions I realized that the AI brainstormed options proposed a really interesting idea which is scoring the effect that our product is having on decision making at the customer. While it’s hard to measure, it’s also the most objective way to assess adoption:

At this point, we added someone to the decision who could help us come up with the metrics we had already so we could evaluate how useful they were in practice for assessing progress. They also outlined how it would be challenging to actually implement a way of assessing the impact of a decision even if every party involved wanted to, because of factors outside our ability to measure.
I find the process of making a decision in the app can be a little nerve wracking despite using it a lot, because:
- When you collaborate on decisions, they become more rigorous by default. It’s hard to ask experienced professionals what they think of something without them poking holes. Often, my hunch is shown to be biased and not thoroughly considered.
- The abilities that we’ve developed into the product. It’s hard to know what the AI is going to come up with, and we’ve found that it consistently does a good job of identifying biases and helping us make predictions about what our options might lead to.
By the next week we had considered the input from the team and from the app’s abilities and were starting to see more clearly which options were the most appropriate according to our criteria. The AI had brainstormed an option to measure the number of teams that use the app per week, partly from inputs from our discussion and partly from its world model, and that ended up as the leading option. But we wanted a way to assess the health within those teams as well, so we had to think about the health within an active team as well.
Here’s where the AI ability recommended we consider a second metric and challenged my bias for only one:
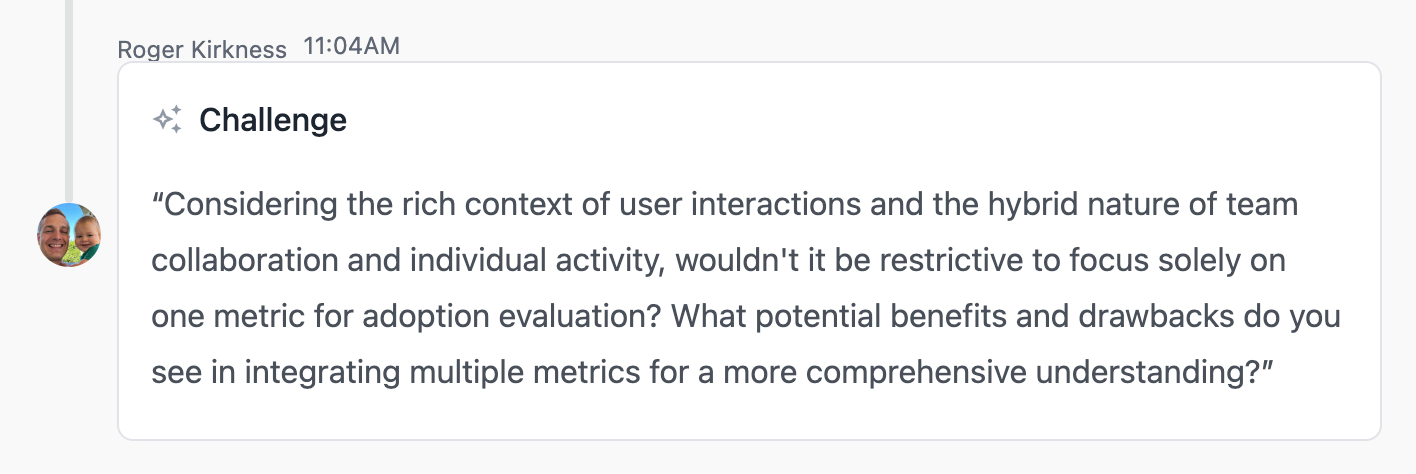
And the options as they existed at the time that we did our final evaluation:
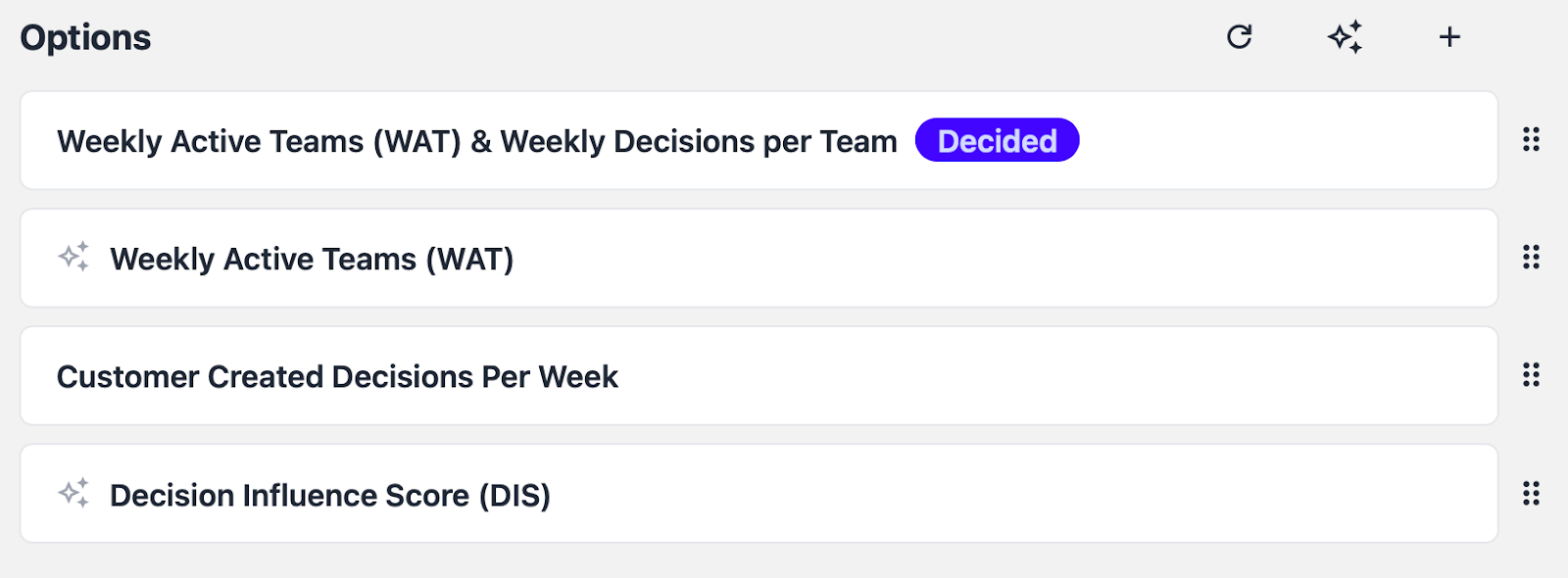
Once we saw the option that I created by combining two AI suggested options (Weekly Active Teams and Decisions Per Team) it became clear to the group that this would probably be the best metric to use. I asked some questions and only got thumbs up responses, implying that I was back in a confirmatory state and ready to decide. The initial hunch I had to measure only the number of decisions as a total amount was replaced with AI driven suggestions. But had the team and I not collaborated on how to implement that option and what we cared about goals and criteria wise, it would not have been able to suggest good options that lead to our eventual choice.
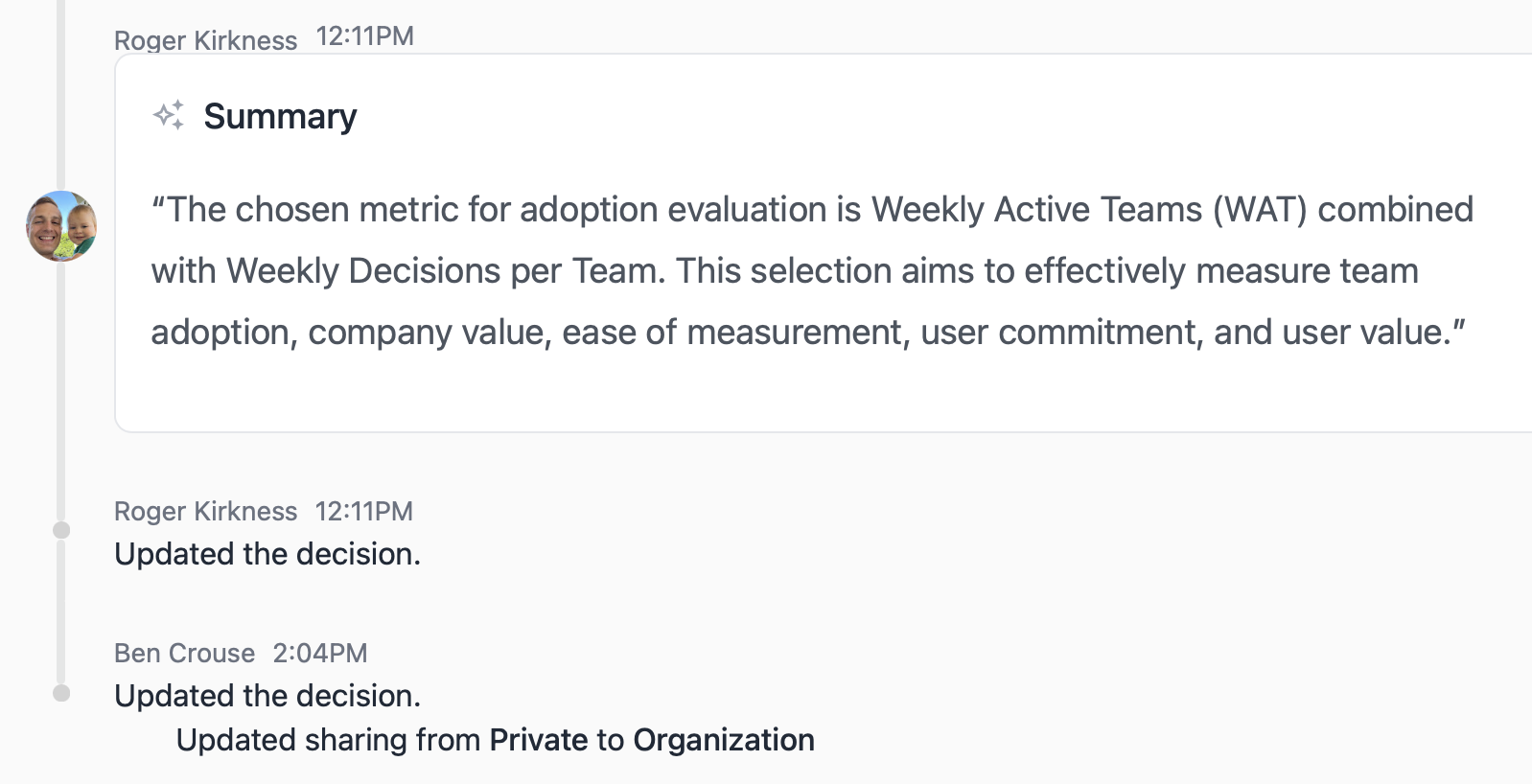
Once I made the decision, the tool gave me a summary I could copy as context into a team email announcing the approach we would take to assessing our R&D progress. A member of our team marked the decision as Public after I had sent that email, so that others on the team could access not only the summary but the entire context of the decision. Now as team members join in the future and have questions about why we use these metrics, we can share the full context of the process with them.
This experience was a turning point for me. It validated the significance of our work and transformed my approach to decision making in my day to day. I shifted from relying heavily on intuition, to embracing collaborative, data-driven decision processes enhanced by AI. This not only improved the quality of our decisions from what we can tell, but also increased my confidence in the potential impact of our tool.
Embracing this new approach has not only enhanced our decision making, but also reaffirmed the potential of our work to revolutionize how businesses operate. By combining human judgment with AI-assisted insights, we're pioneering a path toward more effective and confident decisions. I encourage you to join us on this journey and explore how our tool can benefit your organization.
Note:
If you'd like to browser a static version of this decision, you can access it here.